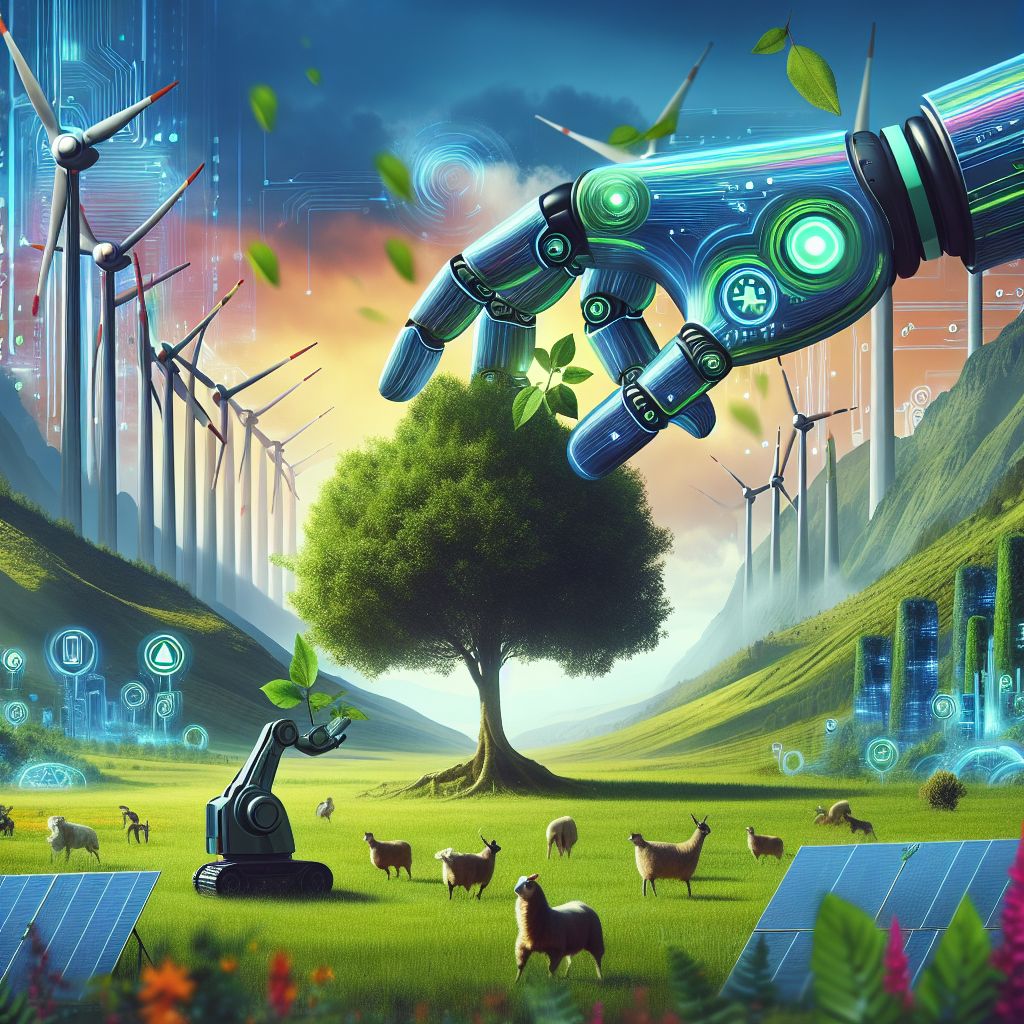
Artificial intelligence is growing at an unprecedented pace, driving innovations in everything from healthcare and finance to entertainment and education. However, training complex AI models, particularly large language models (LLMs), is highly energy-intensive. This immense energy demand contributes to a significant carbon footprint, drawing attention to the environmental impact of AI. As the global climate crisis intensifies, there is a crucial need to address the sustainability challenges posed by AI and seek energy-efficient solutions.
The Importance of Reducing AI’s Carbon Footprint
The carbon footprint associated with AI development stems largely from the energy consumption required for training sophisticated models. There are multiple reasons why reducing this footprint is vital:
1. Environmental Concerns
The environmental cost of AI is significant, with training a single large model estimated to emit as much CO₂ as the lifetime emissions of multiple vehicles. Lowering AI’s energy consumption directly contributes to reducing greenhouse gas emissions and alleviating the environmental burden of technology.
2. Economic Benefits
Energy-efficient AI training is not only beneficial for the environment but also cost-effective. Running data centers, powering advanced GPUs, and maintaining cooling systems all contribute to substantial operational costs. Energy-efficient solutions reduce electricity usage, lowering expenses for companies that deploy AI.
3. Corporate Responsibility and Sustainability
As environmental awareness grows, companies face increasing pressure to adopt sustainable practices. Reducing AI’s carbon footprint aligns with corporate social responsibility (CSR) goals, enhancing brand reputation, improving stakeholder trust, and meeting consumer expectations for green practices.
Current Energy Consumption in AI Training
Training LLMs like GPT-3, BERT, and other similar models requires a massive amount of computational power, translating into high energy consumption. The energy demands for training these models rival those of some traditional energy-intensive industries. Let’s explore some of the main contributors to this energy consumption:
1. Statistics on Energy Usage
The energy usage of AI training varies depending on the model’s size and complexity. For example, training GPT-3 required enough energy to power hundreds of households for a year, resulting in substantial carbon emissions.
2. Factors Contributing to High Energy Consumption
Factors such as data volume, model parameters, and training duration all influence the energy demands of AI training. Larger datasets and models with billions of parameters require more computational resources, leading to higher energy consumption.
3. Comparison with Other Industries
The energy footprint of training large AI models is comparable to, if not higher than, certain traditional industries. For instance, data centers and AI operations may consume as much energy as some small countries, highlighting the urgent need for energy-efficient training practices.
Techniques for Energy-Efficient Training
Reducing the carbon footprint of AI begins with adopting energy-efficient techniques in model training. Here are some of the most promising methods:
1. Model Optimization
Model optimization focuses on reducing the computational load without compromising performance. Techniques such as pruning (removing unnecessary model parameters), quantization (using lower precision numbers), and knowledge distillation (using smaller models) can lead to energy savings while maintaining model quality.
2. Hardware Advancements
Advancements in hardware, particularly energy-efficient GPUs and specialized AI accelerators, significantly contribute to reducing energy consumption. Modern GPUs and TPUs are designed to handle large-scale computations with lower energy costs, making them ideal for sustainable AI training.
3. Algorithmic Improvements
Algorithmic innovations such as efficient neural architectures, better training algorithms, and reduced batch sizes can lead to faster convergence, thereby cutting down on computational requirements and saving energy. Newer training methods are continuously evolving to improve efficiency in AI processing.
Impact of Energy-Efficient Techniques on AI Performance
Energy-efficient training techniques must balance sustainability with performance. Fortunately, many energy-saving methods can be implemented without sacrificing model accuracy or utility. Here’s a closer look at how these techniques impact AI performance:
1. Performance Metrics
Metrics such as model accuracy, latency, and computational throughput are critical in evaluating energy-efficient techniques. In many cases, optimized models achieve nearly identical accuracy compared to their original versions, demonstrating that energy efficiency does not necessarily come at the cost of performance.
2. Case Studies and Examples
Numerous case studies have shown successful implementations of energy-efficient training techniques. For example, companies that have adopted hardware accelerators and optimization algorithms have reported substantial reductions in energy use without compromising model efficacy.
3. Balancing Efficiency and Accuracy
The balance between efficiency and accuracy is a key consideration. Techniques such as model pruning or quantization may reduce energy use while maintaining acceptable accuracy, enabling AI practitioners to strike a balance between sustainability and performance.
Future Directions in Sustainable AI Development
The journey towards a greener AI industry is ongoing, with emerging technologies and practices paving the way for more sustainable AI development:
1. Emerging Technologies
Quantum computing, neuromorphic computing, and optical computing are among the emerging technologies that could drastically reduce the energy demands of AI. These technologies offer the potential for ultra-fast processing with minimal energy usage, promising a more sustainable future for AI.
2. Policy and Regulatory Considerations
Government regulations and industry standards are likely to play a significant role in shaping sustainable AI practices. Regulatory bodies may implement guidelines for energy use in AI development, encouraging companies to adopt sustainable practices.
3. Collaborative Efforts for Sustainability
Collaboration among researchers, industry leaders, policymakers, and environmental organizations is essential for sustainable AI development. Joint efforts in research and development, data sharing, and infrastructure upgrades can drive energy efficiency and reduce AI’s environmental impact.
The carbon footprint of AI is an urgent issue, with the high energy consumption required for training large language models contributing to environmental degradation. However, by embracing energy-efficient training techniques, the AI industry can work toward a more sustainable future. From model optimization to hardware advancements and algorithmic innovations, the path to sustainable AI is within reach.
It’s now up to AI developers, businesses, and policymakers to take action, adopting practices that minimize environmental impact and maximize the benefits of AI technology. Together, we can make AI a powerful force for both technological and environmental progress. The responsibility of reducing AI’s carbon footprint doesn’t rest on one entity alone. Embracing energy-efficient practices will allow us to harness AI’s full potential while safeguarding our planet for future generations. Contact us today.