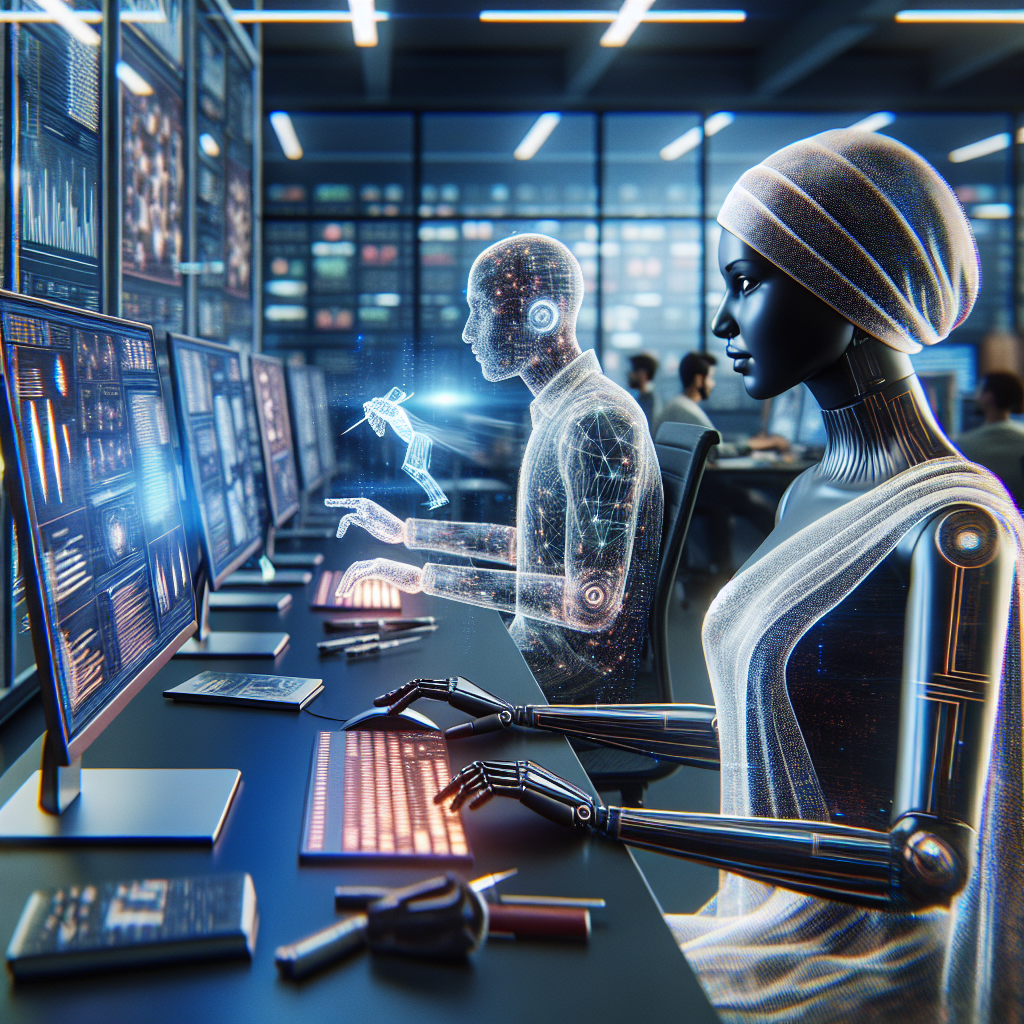
In today’s fast-evolving AI landscape, multi-agent systems (MAS) have emerged as transformative forces in advanced decision-making and predictive analytics. By replicating the interactions of autonomous agents, MAS offer unprecedented insights into complex environments, allowing organizations to assess potential outcomes and devise effective strategies. From finance and healthcare to urban planning and defense, MAS are redefining how industries tackle challenges and uncertainty.
Understanding Multi-Agent Systems (MAS) and Their Role in Decision Support
Multi-agent systems are pivotal for enhancing decision support tools by modeling and predicting outcomes in dynamic, multi-layered environments. Unlike traditional data-driven approaches, which rely mainly on historical data for projections, MAS simulate interactions among autonomous agents—each with unique goals, behaviors, and decision-making capabilities. This setup enables MAS to explore diverse “what-if” scenarios, offering decision-makers a comprehensive view of potential strategies and outcomes.
For example, MAS applications in finance can simulate the behaviors of thousands of independent trading agents, each representing an investor with distinct risk preferences and strategies. By modeling these complex interactions, MAS allow analysts to predict how various economic conditions could influence market dynamics, leading to more robust strategy formulation.
Benefits for Decision-Makers
- Enhanced Scenario Planning: MAS enables visualizing multiple future scenarios, allowing decision-makers to make better-informed choices.
- Improved Accuracy: By capturing intricate agent interactions, MAS provide a holistic view that minimizes the risk of overlooking key variables.
- Risk Management: Decision-makers can identify risks and potential failure points by testing strategies in simulated environments, strengthening resilience.
In short, MAS make decision support tools more dynamic and responsive, empowering organizations to predict and navigate complex environments more effectively.
Advancing Predictive Analytics with Multi-Agent Systems
Predictive analytics, while traditionally reliant on historical data, often struggles to capture the intricate relationships and emergent behaviors within vast, complex datasets. MAS address these limitations by offering simulations that reflect real-world, collective behaviors, thus enhancing the accuracy and reliability of predictive models.
Enhancing Predictive Models with MAS
MAS go beyond static correlations by simulating real-time interactions among agents, such as customers, patients, or suppliers. In healthcare, for example, MAS can model the interactions among patients, healthcare providers, and treatment protocols to predict which treatment approaches may yield the best results. This simulation considers various factors, including patient demographics, medical history, and adherence to treatment, enabling healthcare providers to improve patient outcomes through personalized treatment paths.
In retail, MAS simulations help model customer behaviors, spending habits, and demand trends, allowing businesses to refine inventory management, target marketing strategies, and improve overall customer satisfaction.
Key Predictive Benefits of MAS
- Deeper Pattern Recognition: MAS detect correlations and trends by observing the dynamic interactions between agents, helping businesses make more proactive decisions.
- Greater Forecast Precision: MAS-driven predictive analytics provide highly accurate and detailed forecasts, essential for applications like supply chain management and customer demand forecasting.
- Real-Time Adaptability: Unlike static models, MAS-based predictive analytics adapt to changing circumstances, making them suitable for fast-paced, dynamic industries.
By incorporating MAS into predictive analytics, organizations achieve higher accuracy and generate adaptable, nuanced forecasts that keep pace with rapid industry changes.
Developing Adaptive Strategies with Multi-Agent Systems
In today’s competitive, fast-paced landscape, adaptive strategies—those that evolve in response to changing conditions—are essential for staying relevant. MAS facilitate the development of such strategies by simulating diverse potential outcomes, allowing organizations to experiment with different approaches before real-world implementation.
MAS and Adaptive Strategy Formulation
MAS can simulate how organizations might respond to shifts in regulations, economic fluctuations, consumer preferences, or technological advancements. For instance, logistics companies use MAS to test delivery strategies by modeling interactions among drivers, vehicles, traffic patterns, and even weather conditions. The simulation may include scenarios where specific routes become inaccessible, providing insights on efficient resource reallocation without disrupting service levels.
Retailers also benefit from MAS simulations by testing pricing, discount strategies, and customer service responses, helping them stay agile and responsive in ever-changing markets.
Advantages of MAS in Adaptive Strategy Development
- Scenario Testing: Organizations can test various strategy variations, allowing fine-tuning of responses to a range of potential events.
- Resource Optimization: MAS facilitate efficient resource allocation by predicting how changes might impact outcomes.
- Informed Decision-Making: Decision-makers can anticipate how different factors affect strategies, allowing for more agile, proactive decision-making.
By using MAS to develop adaptive strategies, businesses can respond to challenges and seize opportunities with greater agility, ultimately improving their resilience in volatile markets.
Harnessing Collective AI Behavior
One of the unique aspects of MAS is their ability to study collective AI behavior—emergent patterns that arise when multiple agents interact within a shared environment. This collective behavior offers valuable insights into complex dynamics, helping designers create intelligent systems that operate autonomously while collaborating effectively with others.
Applications of Collective Behavior Analysis
- Crowd Management: MAS simulate crowd behavior in emergency evacuation scenarios, enabling authorities to plan safe evacuation routes and minimize risks.
- Supply Chain Collaboration: In supply chain management, MAS models the dependencies between suppliers, manufacturers, and distributors, helping optimize logistics, reduce bottlenecks, and manage disruptions.
- Social Dynamics and Marketing: MAS simulate social behavior in marketing campaigns by modeling how individuals interact and influence one another, revealing how products may gain popularity or how brand messages spread within a community.
Understanding emergent behaviors within MAS allows organizations to develop strategies that align with real-world dynamics, enhancing system efficiency and scalability.
Integrating MAS with Existing Technologies for Enhanced Capabilities
Integrating MAS with advanced technologies, including machine learning (ML), big data analytics, and cloud computing, extends their capabilities and opens new avenues for application. This integration allows organizations to leverage large datasets, apply advanced analytics, and scale MAS applications seamlessly.
Enhanced Capabilities through Integration
- Machine Learning (ML): ML algorithms enhance MAS by enabling agents to learn from past experiences, which increases the accuracy and reliability of simulations. When combined, ML and MAS allow agents to refine their behavior based on real-world outcomes.
- Big Data Analytics: By integrating with big data analytics, MAS can process vast datasets, uncovering deeper insights into trends and patterns that affect decision-making.
- Cloud Computing: Cloud infrastructure provides MAS with the computational power required to scale simulations, enabling organizations to run concurrent models and complex scenarios efficiently.
In the energy sector, for example, combining MAS with ML helps optimize grid management by analyzing energy consumption patterns and predicting peak times. With cloud-based MAS applications, utilities can better manage demand, reducing costs and enhancing operational efficiency.
Benefits of MAS and Technology Integration
- Scalability: Cloud-based MAS applications can handle extensive simulations and datasets, resulting in more accurate insights.
- Improved Decision-Making: Enhanced data processing capabilities lead to better-informed decisions, allowing organizations to act with confidence.
- Cost Efficiency: Integrating MAS with cloud computing and ML makes simulations more cost-effective by optimizing resources and reducing infrastructure expenses.
Through this integration with modern technologies, MAS evolves from a powerful simulation tool to a comprehensive solution for strategic decision-making.
The Future of Multi-Agent Systems in Decision-Making and Predictive Analytics
As MAS continues to evolve, they offer a robust framework for advancing decision-making and predictive analytics. By harnessing collective AI behavior, MAS empowers organizations to analyze complex interactions, adapt to new challenges, and integrate with cutting-edge technologies. These systems are positioned to shape the future of predictive analytics, enabling industries to navigate complexity with confidence and precision.
Organizations that embrace MAS will be better prepared to thrive in competitive landscapes, leveraging advancements in R&D, optimizing operations, and driving transformative business solutions. Ready to explore the potential of MAS for your business? Connect with us today and discover how MAS can elevate your strategies, helping you stay ahead in a rapidly changing world.
Connect with our expert to explore the capabilities of our latest addition, AI4Mind Chatbot. It’s transforming the social media landscape, creating fresh possibilities for businesses to engage in real-time, meaningful conversations with their audience